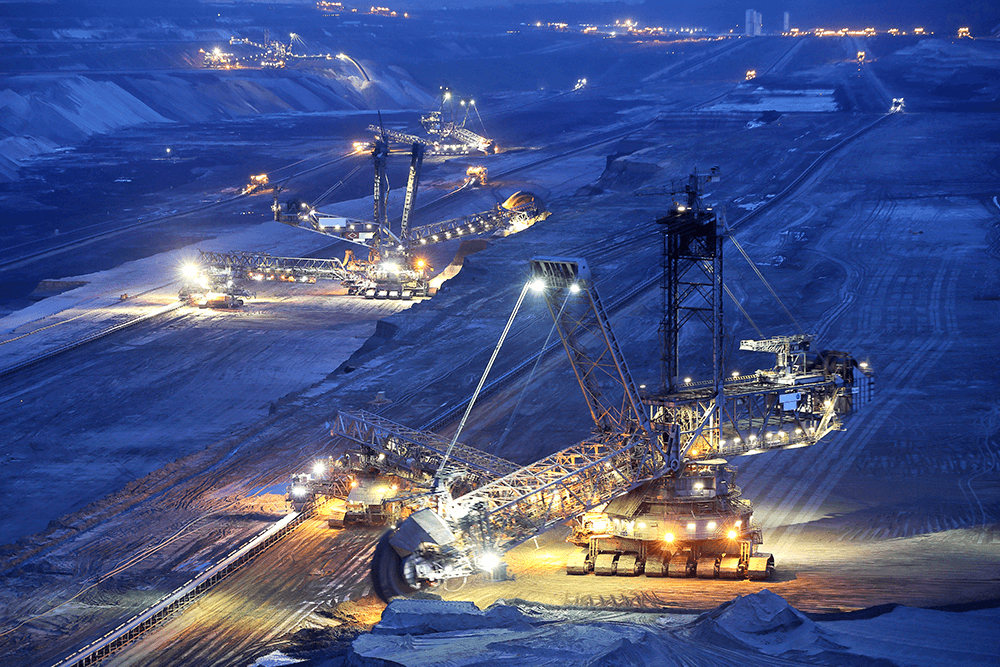
Artificial Intelligence (AI) is no longer a futuristic concept for the mining sector – it has arrived at the forefront of mining technology. From automated haul trucks in Australia’s iron ore mines to AI-driven ore discovery in South America, AI in mining is driving a global digital transformation in mining operations and corporate strategy. Executive leaders of the world’s top mining companies – BHP, Rio Tinto, Vale, Anglo American, Glencore, and others – are championing AI initiatives to boost productivity, safety, and sustainability. “We expect the next big wave in mining to come from the advanced use of digital technologies... next-generation technologies like artificial intelligence, machine learning and data analytics will need to be used to unlock more production and value from our existing mines,” says BHP Chief Technical Officer Laura Tyler. Indeed, the adoption of AI is becoming an imperative across the industry: for mining companies to stay competitive and meet rising sustainability goals, embracing mining technology and automation is not just an option – it’s a necessity.
This AI-driven revolution in mining invites comparison with past transformations. Just as mechanization in the 20th century replaced pickaxes with machines, and digital controls in the late 20th century automated processes, AI represents a new inflection point. The difference? The pace and breadth of change. Virtually no industry has remained untouched by AI, and mining – historically slower to adopt change – is now racing to catch up. The question is not if AI will reshape mining, but how quickly and intelligently mining companies can integrate these tools. Are today’s mining leaders prepared to harness this technology-driven upheaval, or will they be left digging in the past?
Operational AI: From Autonomous Haulage to Smart Exploration
The most visible impact of AI in mining is at the operational level, where it is revolutionizing how mines are run. In open-pit mines and underground operations alike, AI-powered automation and analytics are boosting efficiency, lowering costs, and enhancing safety. Digital transformation in mining is evident in several key operational domains:
-
Autonomous Vehicles and Equipment: One of the earliest AI triumphs in mining was the deployment of autonomous haulage systems. Rio Tinto pioneered this at its Pilbara iron ore mines in Australia as part of its “Mine of the Future” program. Today about 80% of Rio Tinto’s haul trucks in the Pilbara are self-driving, guided by AI for route optimization and obstacle avoidance. These trucks each operate ~700 hours more per year than manned trucks, at 15% lower unit costs. Notably, there have been zero injuries attributed to the autonomous fleet – a stark safety improvement Rio Tinto’s Iron Ore chief executive Chris Salisbury lauded this milestone, highlighting the company’s “pioneering spirit when it comes to adopting revolutionary new technologies which are making the industry safer and more efficient”. BHP has followed suit; over 30% of its haul trucks in Western Australia are automated, and the miner reports significant gains in safety and efficiency from removing human error. Beyond trucks, AI guides autonomous drills, robotic ore-hauling trains, and even driverless water carts across mine sites. The scale is global: Brazilian giant Vale’s Brucutu mine became the first 100% autonomous mine in Brazil in 2019, and Chinese coal operations are undergoing an automation boom with AI and 5G networks – China’s ultramodern Dahaize coal mine achieved a stunning 40% profit margin in 2024 after an “all out” bet on AI-powered automation.
-
AI-Enhanced Mineral Exploration: Finding the next big orebody is like finding a needle in a haystack – a task tailor-made for AI’s data-crunching power. Top miners are leveraging machine learning to analyze geological data faster and more accurately than traditional methods. In May 2023, BHP partnered with Microsoft to deploy AI at the Escondida copper mine in Chile – the world’s largest copper producer – to improve mineral recovery. By feeding real-time sensor data from the processing plant into Azure ML algorithms, the system can recommend adjustments to grinding and flotation parameters, helping operators boost copper output and grade. This collaboration is about more than incremental gains; as BHP notes, global copper production needs to double in the next 30 years to meet demand from electric vehicles and renewable energy. AI is a crucial tool to “unlock more production and value” from increasingly low-grade deposits. Similarly, Vale’s new AI Center in Brazil is accelerating exploration: one initiative uses AI with satellite data to map potential mineralization, and Canadian miner Goldcorp (now part of Newmont) famously used IBM’s Watson to predict gold deposits at its Red Lake mine, reducing discovery time. Even mid-tier players are innovating – Barrick Gold has deployed machine learning via startup Fleet Space’s ExoSphere system for copper exploration in Pakistan’s Reko Diq project, generating 3D subsurface maps to pinpoint ore bodies while minimizing drilling. These AI-driven exploration techniques are helping replenish reserves more efficiently, a critical edge as exploration budgets remain tight.
-
Predictive Maintenance and Processing Optimization: In giant processing plants and critical equipment workshops, AI algorithms are working behind the scenes to prevent failures and streamline operations. Predictive maintenance systems use machine learning to analyze sensor data from trucks, shovels, conveyors, mills, and rail lines, spotting anomalies that indicate impending breakdowns. This enables repairs before equipment fails, avoiding costly unplanned downtime. For example, Evolution Mining in Australia uses AspenTech’s AI-powered Mtell system at its Mungari gold mine to forecast equipment failures and has mitigated safety risks by catching faults early. Vale credits its AI programs with significantly extending equipment life: at its Salobo copper mine, AI analysis of haul truck data boosted tire life by 30% in one year, saving millions. In processing plants, AI-based control systems constantly fine-tune grinding and flotation settings for maximal metal recovery. Rio Tinto employs AI in automated iron ore rail control and even in a fully automated on-site laboratory, achieving record throughput. Anglo American has implemented digital twins – virtual AI-driven replicas of equipment and processes – at its new Quellaveco copper mine in Peru. These digital models “learn” how the plant should behave and recommend adjustments to avoid failures, optimize energy use, and reduce downtime. According to Anglo, Quellaveco’s digital twin system (adapted from NASA technology) is helping make it one of the most efficient and safest operations, truly a “100% digital mine”.
-
Safety and Monitoring: AI’s watchful eyes are improving mine safety in ways previously impossible. Computer vision algorithms monitor real-time video feeds to detect hazards (like people or vehicles in restricted zones) and automatically enforce exclusion zones. Wearable sensors combined with AI alert supervisors if a worker shows signs of fatigue or heat stress, allowing intervention before incidents occur. AI-driven risk prediction models analyze myriad data – ground movement readings, equipment vibrations, weather – to warn of slope failures or rock bursts in underground mines, enhancing worker protection. Some governments are even using AI and satellite imagery to identify illegal mining and deforestation from tell-tale landscape changes. The cumulative effect is a step-change in safety: fewer human hours in harm’s way thanks to autonomous systems, and smarter prevention strategies. As one former industry technology director observed, the main hurdle now is trust – miners must trust AI systems enough to act on their warnings. “The main challenge to AI adoption in the mining industry is trust. In this high-stakes environment, miners are hesitant to fully depend on AI-generated empirical models. A hybrid approach, an ‘open loop control,’ which incorporates necessary human intervention, is seen as the path forward,” advises Keith Flynn, former Senior Director at AspenTech. In practice, this means AI can take over routine decisions, but humans remain in the loop for oversight – a partnership of machine precision and human judgment aimed at zero harm operations.
Strategic AI: From Boardroom to ESG Reporting
AI in mining isn’t only about robots and autonomous vehicles; it’s also increasingly influencing corporate strategy, finance, and ESG and AI initiatives at the executive level. In the boardroom and back office, mining leaders are harnessing AI for data-driven decision-making that spans from financial modeling to workforce planning and sustainability compliance.
Financial Modeling & Decision Support: The volatility of commodity markets and complexity of global operations make mining a data-intensive business. Today’s CFOs and strategists use AI-driven analytics to navigate this complexity. Machine learning models ingest historical production, cost, and market data to produce more accurate forecasts for commodity prices and demand, helping companies plan capital expenditures and project developments with greater confidence. AI-powered financial modeling can rapidly run scenarios (“What if iron ore prices drop 20%?” or “How would a carbon tax affect margins?”) that inform strategic choices. Digital transformation in mining finance means less time spent on spreadsheets and more on insights: in many industries, AI has improved forecasting accuracy and cut data processing time significantly. However, mining CFOs remain pragmatic – ultimately, if an AI’s prediction is wrong, the accountability falls on the company, not the algorithm. Thus, many firms adopt AI as an augmented advisor: it highlights patterns and anomalies human analysts might miss, while executives apply experience and caution to the final call.
Workforce Planning & Automation of Administration: Managing a large, remote workforce and complex supply chains is another area ripe for AI assistance. Mining HR departments are experimenting with AI to optimize workforce planning – for example, algorithms that predict attrition or training needs by analyzing workforce data, or scheduling systems that use AI to allocate shifts in mines for optimal productivity while minimizing fatigue risk. Routine administrative processes are also being streamlined through AI and robotic process automation (RPA): from processing invoices to compliance paperwork, bots can handle repetitive tasks faster and with fewer errors. One major iron ore producer noted that automating forms and workflows has increased accuracy and freed staff for higher-value work. Some companies are even tapping generative AI to draft routine reports or analyze legal and regulatory documents. The result is a leaner, more responsive organization. Crucially, mining firms recognize that the “future of work” in mining will be shaped by AI – requiring new skill sets. Progressive companies are investing in upskilling programs so that engineers and analysts can work effectively alongside AI tools. “We understand the impact technology and the future of work will have… we have to be a catalyst in those local communities for new jobs,” said former Anglo American CEO Mark Cutifani, emphasizing that miners must create new roles and retrain workers as automation expands. In essence, AI is influencing organizational strategy: companies that proactively reskill employees and redesign jobs can both soften the blow of automation and unleash human creativity in partnership with machines.
ESG Reporting and Sustainability: Perhaps nowhere is AI’s influence more mission-critical to mining’s future than in the realm of Environmental, Social, Governance (ESG) performance. Mining companies face intense scrutiny to reduce their environmental impact, ensure worker safety and diversity, and uphold ethical governance. Here, AI is becoming an invaluable tool. AI-driven analytics are streamlining ESG data collection and reporting, turning what used to be an annual compliance exercise into a continuous improvement cycle. For instance, sensors across a mine measure water usage, emissions, and energy consumption in real time; AI platforms aggregate this data and flag anomalies or inefficiencies instantly. Companies using AI for ESG data management have reported up to a 40% reduction in data processing time and 30% increase in reporting accuracy, allowing faster and more reliable disclosure of sustainability metrics. This improves transparency with investors and regulators – a key governance concern – and helps executives spot environmental issues early (such as an unexpected spike in water draw or dust levels) to take corrective action.
AI is also directly aiding sustainability initiatives on the ground. In pursuit of decarbonization, miners use AI to optimize haul truck routes and grinding circuit efficiency, cutting fuel and electricity use. Fortescue Metals Group, for example, employs AI in its scheduling and control systems at its remote operations center “The Hive,” orchestrating 200 autonomous haul trucks to minimize idle time and fuel burn. AI modeling can identify energy savings opportunities in processing plants or suggest alternative, less carbon-intensive methods. On the social front, AI-enhanced safety systems (discussed earlier) support the “S” in ESG by reducing workplace accidents and protecting employees. Even tailings dam monitoring – a critical safety and environmental issue – is being improved by AI algorithms that analyze sensor data for early warning signs of instability, aiming to prevent disasters.
Governance-wise, the use of AI raises its own questions: How to avoid algorithmic bias? How to ensure AI decisions are transparent and ethical? Regulators are moving to address this – the EU’s AI Act, for example, introduces strict requirements for high-risk AI systems. Mining companies are beginning to factor AI governance into their broader corporate governance frameworks. Those that get it right see AI as a powerful ally to their ESG commitments – helping them become safer (social), greener (environmental), and more transparent (governance). As Vale’s Digital Transformation Director, Afzal Jessa, summed up: “Artificial Intelligence has the potential to generate value for all business areas of the company… we’re taking another important step towards digital transformation to increase productivity and operational efficiency, achieve the highest levels of health and safety, improve our financial performance and drive innovation”. In other words, AI and ESG go hand in hand: the technology is enabling better environmental stewardship and safety, which in turn secures mining’s social license to operate in the long run.
Lessons from Past Transformations
Mining has undergone major technological shifts before – mechanization, electrification, and the advent of computers all transformed how mines operate. What makes the AI revolution similar or different? One parallel is the productivity leap: much as mechanical shovels vastly out-dug manual labor, AI-driven automation promises a step change in output and cost efficiency. Rio Tinto’s experience with autonomous trucks (15% cost reduction, zero injuries) echoes the productivity vs. safety win-win that mechanization achieved decades ago. Another parallel is the initial skepticism and workforce impact. Just as miners once feared that mechanization would eliminate jobs (only for new, different jobs to eventually emerge), today there are concerns that AI and robots could displace jobs or deskill roles. The industry’s response has been to proactively manage this transition: retrain operators as remote vehicle controllers or data analysts, and engage communities about the changing nature of mine employment. History suggests that mining companies that embraced new technology early often gained a competitive edge – for example, early adopters of modern earthmoving equipment dominated mid-20th century mining. The same is likely with AI: companies that invest now in AI capabilities can “create the future” of mining, rather than be victims of it, to paraphrase Cutifani.
However, AI is also different from past innovations in its breadth of impact. Mechanization affected the physical extraction process; AI has the potential to touch every part of a mining business, from how we discover minerals to how we market them. This creates a steeper learning curve and demands a more holistic change management. Integration costs can be high – retrofitting AI into old equipment and training staff – but these costs are expected to decline as the technology matures and scales. Early adopters often shoulder higher upfront costs, much like early electrification projects did, but over time laggards risk falling behind as AI-driven efficiencies become the new normal. It’s instructive to recall how slow adoption of digitization (like real-time data systems) left some miners struggling in the 2000s, whereas those who digitized gained in productivity and cost control. AI is poised to have an even larger impact. The digital transformation in mining through AI could very well separate the leaders from the rest, just as past leaps in technology have done.
Future Outlook: Opportunities and Strategic Implications
What does the AI-enabled future hold for the mining sector? Executives are envisioning mines that are virtually self-driven, with humans overseeing a fleet of intelligent machines from remote integrated operations centers. In this “mine of the future,” AI systems will continuously optimize the entire value chain: automated drills will adjust patterns based on orebody AI models; smart trucks and conveyors will synchronize to feed crushers with minimal energy waste; processing plants will auto-tune themselves in real time; and supply chains will respond dynamically to market demand signals. Some companies are already trialing such concepts – for instance, Teck Resources partnered with tech firms to create an immersive AI-powered digital twin of its operations for real-time scenario planning. It’s plausible that within the next decade, we will see the first operations that approach a true “autonomous mine” – where human intervention is minimal and strategic rather than operational.
From a strategic standpoint, AI could alter the competitive balance in mining. Those with access to cutting-edge AI technology and the data to fuel it will gain a significant advantage, while others risk being left behind. Already, AI-focused startups like KoBold Metals (backed by Bill Gates and others) are emerging to fill gaps, using AI to find battery metals and attracting huge investments. Traditional mining companies might partner with such tech firms or develop in-house AI divisions to avoid ceding ground. We may also see more cross-industry collaboration – with tech giants (Microsoft, Google) and industrial firms (Caterpillar, Komatsu) co-developing AI solutions tailored to mining. In fact, mining is becoming a proving ground for industrial AI applications given its scale and variability of conditions. This trend could accelerate innovation but also means mining companies must be open to new partnership models and even new business models (for example, selling their proprietary AI solutions to peers or diversifying into technology services).
Crucially, AI is opening new frontiers in sustainability and resource development. Generative AI models are being used in research to discover novel, more efficient battery materials – a development that could influence long-term demand for certain minerals. In exploration, AI might enable mining of currently uneconomic deposits by identifying smart ways to extract value. And in processing, we might see AI help develop techniques to economically recycle minerals from waste (tailings reprocessing) or from end-of-life products, supporting the circular economy. These are strategic shifts that could extend mining companies’ value chains beyond traditional extraction.
Yet with all these opportunities, mining leaders must keep a sober eye on risk. The hype around AI is substantial – will these high expectations be met, or are we facing an AI bubble that could burst? The answer will depend on execution. Issues like cybersecurity (protecting the vast data and preventing AI system hacks) and regulatory compliance (as AI gets regulated) need strong governance. Ethical considerations are also front and center. As AI takes over tasks, companies face questions about how to redeploy or compensate displaced workers – echoing social responsibility commitments. Bias in algorithms must be checked to ensure, for example, that an AI HR tool isn’t inadvertently disadvantaging certain groups, or an AI-driven community engagement program isn’t missing key human nuances. In essence, the strategic implication is that deploying AI is not just a technical endeavor but a leadership challenge: it requires vision to invest early, discipline to manage risks, and empathy to bring people along.
Conclusion: Leading the Smart Mining Revolution
The stage is set for AI to become as integral to mining as drills and bulldozers. Sam Altman, CEO of OpenAI, noted that the development of AI – including powerful generative AI – is only accelerating, with even more advanced “superintelligence” likely within the next decade. In parallel, three of the top ten emerging technologies of 2024 identified by the World Economic Forum are rooted in AI. The writing on the wall is clear: regardless of whether a particular mining company has fully embraced AI yet, this disruptive innovation is rapidly gaining ground and reshaping industry norms. For an industry that has historically been slow to change, the AI in mining wave presents a choice – ride it proactively or risk being swept aside by more agile competitors.
Executive leaders reading this are tasked with a delicate balance. On one hand, they must maximize the gains AI offers: higher productivity, lower costs, improved safety, and enhanced ESG outcomes. On the other, they must manage the perils: integration costs, talent gaps, ethical pitfalls, and the ever-present uncertainty of new technology. As pressures mount – from the need to meet decarbonization targets and critical mineral demand, to expectations for safer workplaces and stronger governance – the incentive to leverage AI only grows. In fact, a combination of profit imperatives and ESG pressures will likely compel the industry to adopt and adapt, integrating AI across mining operations in the coming years.
Will AI in mining ultimately live up to its promise? The evidence so far is encouraging: smarter mines are already more productive, and early adopters are reporting tangible benefits. The skeptics are being proven wrong one haul truck, drill, and dataset at a time. But success is not guaranteed for all. The next decade will reveal which mining companies can evolve into truly “smart mines” and which struggle with the transformation. It’s a time of opportunity and introspection. As one industry expert poignantly asked, will the much-discussed AI boom deliver real returns or end up as another tech hype cycle? For mining’s executive leaders, the charge is to ensure it’s the former. By fostering a culture of innovation, investing in people and systems, and keeping a clear focus on strategic objectives and sustainability, they can harness AI to build mining companies that are not only more profitable, but also safer, cleaner, and more responsible. The mining industry is at the dawn of an AI-powered era – those with the vision and discipline to lead this change will define the future of mining.